
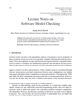
Faced with the disruption generated by the COVID-19 pandemic, the advent of enforced and exclusive online learning presented a challenging opportunity for researchers worldwide, to quickly adapt curricula to this new reality and gather electronic data by tracking students’ satisfaction after attending online modules. Many researchers have looked into the subject of student satisfaction to discover if there is a link between personal satisfaction and academic achievement. Using a set of data, filtered out of a statistical analysis applied on an online survey, with 129 variables, this study investigates students’ satisfaction prediction in a first-semester Mechanical Engineering CAD module combined with the evaluation and the effectiveness of specific curriculum reforms. A hybrid machine learning model that has been created, initially consists of a Generalized Linear Model (GLAR), based on critical variables that have been filtered out after a correlation analysis. Its fitting errors are utilized as an extra predictor, that is used as an input to an artificial neural network. The model has been trained using as a basis the 70% of the population (consisting of 165 observations) to predict the satisfaction of the remaining 30%. After several trials and gradual improvement, the metamodel’s architecture is produced. The trained hybrid model’s final form had a coefficient of determination equal to 1 (R = 1). This indicates that the data fitting method was successful in linking the independent variables with the dependent variable 100 percent of the time (satisfaction prediction).