
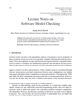
Detecting anomalies in the traffic of computer networks is an important step in protecting and countering various types of cyber attacks. Among the many methods and approaches for detecting anomalies in network traffic, the most popular are machine learning methods that allow one to achieve high accuracy with minimal errors. One of the ways to improve the efficiency of anomaly detection using machine learning is the use of artificial neural networks of complex architecture, in particular, networks with long short-term memory (LSTM), which have demonstrated high efficiency in many areas. The paper is devoted to the study of the capabilities of LSTM neural networks for detecting network anomalies. It proposes using LSTM neural networks to detect network anomalies caused by cyber attacks to bypass Web Application Firewall vulnerabilities that are very difficult to detect by other means. For this purpose, it is proposed to use LSTM in conjunction with an autoencoder. The issues of software implementation of the proposed approach are considered. The experimental results obtained using the generated dataset confirmed the high efficiency of the developed approach. Experiments have shown that the proposed approach allows detecting cyber attacks in real or near real time.