
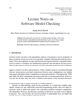
The area of knowledge representation and reasoning is concerned with simulating intelligent behaviour in automated agents using explicit, formal representations of domain or evidential knowledge. We consider rules with exceptions, called conditionals, within the broader context of inductive reasoning for representing and reasoning with uncertain knowledge. Inductive reasoning techniques transform a finite and incomplete knowledge base into a complete representation of the knowledge and beliefs of the agent operating with this knowledge base.
We employ Spohn’s ranking functions that assign degrees of implausibility to the interpretations of the propositional language used to formulate the defeasible rules representing uncertain knowledge. A special kind of ranking functions, the c-representations introduced by Kern-Isberner, will take a central role in our investigations.
In particular, we investigate how reasoning using sets of ranking models can be understood, researched, implemented, and optimised. We define modes of inference that, together with the choice of the actual set of ranking models, give us two dimensions along which to position inference relations induced by conditional knowledge bases. To understand the relationships among these inference relations better, we introduce a classification of qualitative conditionals with respect to semantic properties of conditionals. The idea of redundant conditionals proposed in the literature is elaborated upon, while investigating properties of c-inference relations. We show that adding or removing redundant conditionals from knowledge bases can change the induced c-inference relations, therefore highlighting that explicit and inferred information is considered different within the framework of c-representations. We exploit our classification of conditionals to optimise the task of calculating complete inference relations, also employing a novel data structure for representing inference relations.
To optimise the task of answering queries with respect to sets of c-representations, we introduce a compact representation of conditional knowledge bases, designed to capture the computational complexity of answering queries. To make implementing c-inference practical, we introduce a finite domain variant of the constraint satisfaction problem used to calculate c-representations and characterise kinds of upper bounds used in solving it. We investigate formal properties of inference relations defined over sets of ranking models by considering inference postulates proposed in the literature and by presenting an approach for experimentally investigating inference systems. We show for instance that credulous and weakly skeptical inference over arbitrary sets of ranking models satisfies the properties (REF), (LLE), (RW), (VCM) and (WAND). We also show that c-inference relations satisfy the property weak rational monotony (WRM), which is not generally satisfied by inference relations defined over arbitrary sets of ranking models. Finally, we present an implementation, called InfOCF-Lib, featuring all proposed techniques, algorithms, and data structures in form of an easy to use Java library.