
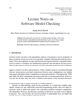
Concerning the problem that the current facial expression analysis based on convolutional neural network (CNN) only uses the features of the last convolutional layer but the recognition rate is not high, this paper proposes the use of sub-deep convolutional layer features and builds a CNN model which fuses the features of multi-layer convolutional layers. The model uses a CNN for feature extraction and saves the deepest feature vectors and sub-deep feature vectors of the expression images. The sub-deep feature vector is used as the input of the multilayer CNN established in this paper. The processed fourth convolution layer feature is fused with the deepest feature previously saved to perform facial expression analysis. Experiments are performed on FERPLUS dataset, Cohn-Kanade dataset (CK+) and JAFFE dataset. The experimental results show that the improved network structure proposed in this paper can capture richer feature information during facial expression analysis, which greatly improves the accuracy of expression recognition and the stability of the network. Compared with the original CNN-based facial expression analysis using only the last layer of convolution layer features, using multi-layer fusion features on three kinds of datasets can improve the expression recognition rate by 33.3%, 2.3% and 22%, respectively.