
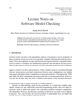
Clinical Algorithms (CAs) are obtained from Clinical Practice Guidelines (CPGs) using all the healthcare knowledge available to assist patients that suffer from one or several diseases. For some diseases CAs can be explicitly given, while for others we have to use other mechanisms for their generation. Explicitly given CAs use to be atemporal. To provide the time dimension of CAs it is necessary to obtain temporal knowledge from physicians or by some other mechanism. Often, physicians have difficulties in providing temporal knowledge or the knowledge engineering mechanism and tools used are difficult to apply or extremely time consuming. However, as data saved in clinical databases are time dependent, they can be used to implicitly obtain temporal constraints for CAs. We have identified two sorts of temporal constraints (micro- and macro-temporality) and have proposed an approach to generate micro-temporalities that complements previous works. We have decided to use the SDA (state-decision-action) formalism for CA representation. The generated micro-temporality constraints are introduced in the SDA representation of a particular CA. As the final CAs have a time dimension, they are no longer atemporal, which helps physicians making temporal predictions in healthcare procedures.