
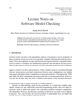
Social robots are embodied agents that continuously perform knowledge-intensive tasks involving several kinds of information coming from different heterogeneous sources. Providing a framework for engineering robots’ knowledge raises several problems like identifying sources of information and modeling solutions suitable for robots’ activities, integrating knowledge coming from different sources, evolving this knowledge with information learned during robots’ activities, grounding perceptions on robots’ knowledge, assessing robots’ knowledge with respect humans’ one and so on. Semantic Web research has faced with most of these issues and can provide robots with the means for creating, organizing, querying and reasoning over knowledge. In fact, Semantic Web standards allow to easily integrate data generated by a variety of components, thus enabling robots to make decisions by taking into account knowledge about physical world, data coming from their operating environment, information about social norms, users’ preferences and so on. Semantic Web technologies provide flexible solutions that allow to extend and evolve robots’ knowledge over time. Linked (Open) Data paradigm (a result of research in the Semantic Web field) lets to easily reuse (i.e. integrate with robots’ knowledge) existing external datasets so to bootstrap a robot’s knowledge base with relevant information for its activities. Linked Data also provides a mechanism that allows robots to mutually share knowledge. Existing solutions for managing robots’ knowledge only partially exploit the potential of Semantic Web technologies and Linked Data. This thesis introduces a component-based architecture relying on Semantic Web standards for supporting knowledge-intensive tasks performed by social robots, and whose design has been guided by requirements coming from a real socially assistive robotic application. All the components contribute to and benefit from the knowledge base which is the cornerstone of the architecture. The knowledge base is structured by a set of interconnected and modularized ontologies which are meant to model information relevant for supporting robots in their daily activities. The knowledge base is originally populated with linguistic, ontological and factual knowledge retrieved from the Linked Open Data. The access to the knowledge base is guaranteed by Lizard, a tool that provides software components with an API for accessing facts stored in the knowledge base in a programmatic and object-oriented way. This thesis also introduces two methods for engineering knowledge needed by robots: (i) A novel method for automatically integrating knowledge coming from heterogeneous sources with a frame-driven approach. (ii) A novel empirical method for assessing foundational distinctions over Linked Open Data entities from a common sense perspective (e.g. deciding if an entity inherently represents a class or an instance from a common sense perspective). These methods realize two tasks of a more general procedure meant to automatically evolve robots’ knowledge by automatically integrating information coming from heterogeneous sources, and to generate common sense knowledge by using Linked Open Data as empirical basis. Feasibility and benefits of this architecture have been assessed through a prototype deployed in a real socially assistive scenario, whose this thesis presents two applications and the results of a qualitative and quantitative evaluation.