
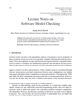
Specific predictive models for diabetes polyneuropathy based on screening methods, for example Nerve conduction studies (NCS, can reach up to AUC 65.8 – 84.7 % for the conditional diagnosis of DPN in primary care. Prediction methods that utilize data from personal health records deal with large non-specific datasets with different prediction methods. Li et al. utilized 30 independent variables, which allowed to implement a model with AUC = 0.8863 for a Multilayer perceptron (MLP). Linear regression (LR) based methods produced up to AUC = 0.8 %. This way, modern data mining and computational methods can be effectively adopted in clinical medicine to derive models that use patient-specific information to predict the development of diabetic polyneuropathy, however, there still is a space to improve the efficiency of the predictive models. The goal of this study is the implementation of machine learning methods for early risk identification of diabetes polyneuropathy based on structured electronic medical records. It was demonstrated that the machine learning methods allow to achieve up to 0.7982 precision, 0.8152 recall, 0.8064 f1-score, 0.8261 accuracy, and 0.8988 AUC using the neural network classifier.