
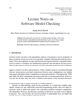
The use of statistical body shape models (SBSM) offers the possibility to generate a realistic full body shape with a limited number of measures/predictors such as traditional anthropometric dimensions, surface landmarks etc. The purpose of the present work is to explore the possibility to create a personalized surface model with a small set of easily measurable parameters, and to compare the quality of SBSM-based prediction in function of predictors. A sample of 164 full body scans in a standing posture from European and Chinese males were selected based on stature and BMI. After cleaning the raw scans, a non-rigid mesh deformation method was used to fit a customized template onto scans. Then, a principal component analysis (PCA) was performed to build SBSM with different set of predictors, including anthropometric dimensions, landmarks’ coordinates, postural parameters. The partial least square regression was used to take into account correlated nature between predictors. As statistical models cannot match the target values of predictors, an optimization was further proposed for better matching targets while not deviating too much from the initial prediction by statistical regression. A leave-one-out (LOO) procedure was used to evaluate the quality of SBSM with different set of predictors.