
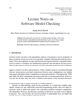
When a conversational group is approached by a newcomer who wishes to join it, the group may dynamically react by adjusting their positions and orientations in order to accommodate it. These reactions represent important cues to the newcomer about if and how they should plan their approach. The recognition and analysis of such socially complaint dynamic group behaviors have rarely been studied in depth and remain a challenging problem in social multi-agent systems. In this paper, we present novel group behavior recognition models, attention-based and graph-based, that consider behaviors on both the individual and group levels. The attention-based category consists of Approach Group Net (AGNet) and Approach Group Transformer (AGTransformer). They share a similar architecture and use attention mechanisms to encode both temporal and spatial information on both the individual and group levels. The graph-based models consist of Approach Group Graph Convolutional Networks (AG-GCN), which combine Multi-Spatial-Temporal Graph Convolutional Networks (MST-GCN) on the individual level and Graph Convolutional Networks (GCN) on the group level, with multi-temporal stages. The individual level learns the spatial and temporal movement patterns of each agent, while the group level captures the relations and interactions of multiple agents. In order to train and evaluate these models, we collected a full-body motion-captured dataset of multiple individuals in conversational groups. Experiments performed using our models to recognize group behaviors from the collected dataset show that AG-GCN, with additional distance and orientation information, achieves the best performance. We also present a multi-agent interaction use case in a virtual environment to show how the models can be practically applied.