
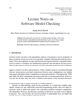
Edge and fog computing mainly deal with Internet of Things (IoT). Practically, problems related to remote sensors or devices are typically where edge computing and fog computing incorporate. Thermal comfort of urban open spaces is one of the most important topics in the field of edge and fog computing. It is necessary to fulfill the demands for more pleasant thermal comfort in urban planning and design new urban open spaces, as well as reviewing and improving the existing ones. The thermal comfort of urban open spaces is variable since it depends on climatic parameters and other influences, which are inconstant throughout the year, as well as during the day. Therefore, the prediction of thermal comfort is significant in order to enable planning of the usage time of urban open spaces. This research aims to develop an Improved Cuckoo Search (ICS) algorithm for forecasting physiological equivalent temperature (PET) values one hour ahead. Usually, the parameters of Cuckoo Optimization Algorithms (COAs) are kept constant, which may lead to efficiency reduction. To cope with this issue, a proper strategy for tuning the parameters is presented. Moreover, the generation of laid eggs is done by implementing the cross-over operator of a Genetic Algorithm (GA). Then, it is employed to train feed forward neural networks for PET prediction. Finally, the performance of the proposed algorithm is compared to the state-of-the-art; i.e., traditional COA and GA. Our simulation results demonstrate the effectiveness of the proposed algorithm for about a 93% compliance rate.