
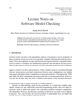
Cyber Physical System (CPS) is one of the key technologies of industry 4.0. CPS is an integrated system that combines computing, sensors and actuators. CPS is controlled by computer-based algorithms that integrates people and internet. However, the performance of CPS is limited by its computational complexity. How to implement CPS with less computational complexity in a real time performance remains an important issue. Therefore, the study attempts to establish a CPS based on machine learning to reduce its complexity and improve applicability. This study applies a Fault Detection and Classification system (FDC) with machine learning method in the virtual subsystem of CPS. This study consists of two steps. Based on the data collected from sensors of CPS, Random Forest (RF) is used to figure out key factors that reduces the data dimension for less computational complexity. The process engineers will know which factors are causing the machine fails. The second step is to establish a time series model which bases on Long Short-Term Memory (LSTM) network to achieve real time monitoring of the machine. Before the machine fails, FDC will alarm that allow the engineer to adjust parameters of machines to avoid machine fails. The study in order to achieve the goals of predicting machines fails, figuring out key factors, predicting maintenance and increasing the productivity.