
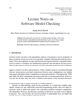
In this paper, we explore a Convolutional Neural Network (CNN) based architecture that learns the audio cues to predict the Big Five personality traits score of a speaker. Our model takes advantage from a pre-trained model on a large database for audio event recognition (AudioSet). The pre-trained model has been fine-tuned on the First Impression Dataset to obtain an audio representation for personality trait recognition. In addition, we interpret our model and generate the visual correlation between the model parameters and learned representations by exploring the Class Activation Maps (CAM). Our results show that our interpretable CNN architecture slightly outperforms, in terms of accuracy, previous methods based on hand-crafted features.We also explore a CNN model trained from scratch which takes as input the raw audio data in the frequency domain, finding some discriminative frequency patterns for each personality trait. The interpretability part reveals the inter-mechanism of the model, showing that some frequency bands are more discriminative for personality trait recognition than others.