
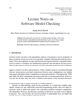
Importance sampling is a Monte Carlo method that samples from an alternative distribution, the proposal distribution. It focuses the sampling process in the interesting parts of space reducing the variance. The efficiency of importance sampling grows as the proposal distribution resembles the original probability distribution (with thicker tails). A reasonable idea for selecting an appropriate proposal distribution is to use a variational approach. Variational inference selects, from a given family, the distribution which minimizes the divergence to the distribution of interest. In this study, we identify the Rényi projection of order 2 as the one that leads to minimum variance estimators. However, its high computational cost pushes us to consider the standard variational approach (I-projection, which considers the reverse Kullback-Leibler divergence), with limited performance in the general case. We finally show that softening the I-projection is an interesting practical work-around to take the best from both projections. The whole discussion is supported by an empirical study in a simple domain of discrete multivariate distributions.