
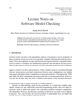
Mapping the microscopical organization of the human cerebral cortex provides a basis for multimodal brain atlases, and is indispensable for allocating functional imaging, physiological, connectivity, molecular, or genetic data to anatomically well specified structural entities of human brain organization at micrometer resolution. The analysis of histological sections is still considered a “gold standard” in brain mapping, and compared with other maps, e.g. from neuroimaging studies [1]. But while the spatial patterns of neuronal cells are inherently three-dimensional, such microscopic analysis is usually performed in individual 2D sections. Here we propose an HPC-based workflow that aims to recover the three-dimensional context from a stack of histological sections stained for neuronal cell bodies, imaged under a light microscope. Our aim is to align image data in consecutive sections at the micrometer resolution, where the texture is dominated by small objects like cell bodies, that often do not extend across sections. Therefore we cannot apply classical intensity-based image registration, where similarity of neighboring images is optimized at the pixel level. Our main contribution is a procedure to explicitly detect and match vessel-like structures in the brain tissue, guiding a feature-based image registration algorithm to 3D reconstruct regions of interest in the brain and recover the distribution of neuronal cells. To replace erroneous information in corrupted tissue areas, we further propose a simple predictive algorithm which generates realistic cell detections by learning from intact tissue parts in the local surroundings.