
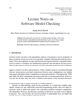
Thermal infrared tracking (TIR) is able to track objects in dark environments such as night. It can be used mainly for surveillance and rescue for surveillance cameras at night. While the development of automatic driving is progressing in recent years, we believe that thermal infrared tracking can contribute to the improvement of safety even in places with few streetlights. However, unlike normal visual object tracking, thermal infrared tracking itself has some problems. In this paper, we propose an algorithm for improving the accuracy by selecting the optimal feature map for each sequence using Kullback-Leibler divergence (KLD) amount for ensemble tracking using the powerful expression ability of convolutional neural network (CNN). Using KLDs from response maps obtained from an ensemble tracker with multi-layer convolutional features in thermal infrared tracking (MCFTS), we determine the CNN filter most involved in creating the response map. By adjusting the bias value corresponding to these filters and learning the filter, it is possible to create a tracker corresponding to the sequence each time. In order to evaluate the performance of the tracker and conventional tracker which applied the proposed algorithm, we experimented with the thermal infrared tracking benchmark of VOT-TIR2016. We also compared the 24 types of trackers that were evaluated in the thermal infrared tracking benchmark. The experimental results demonstrate that the proposed tracker achieves effective and promising performances with some sequences.