
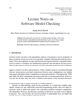
This paper compares two metaheuristic neural networks (ANNs) models, Bat algorithm neural network (BANN) and Bat optimisation neural network (BatNN) for spatial downscaling of long term precipitation. For BANN, model parameters for both pulse rate (R) and loudness (A) are fixed as 0.5. Whilst, R and A parameters for BatNN will dynamically self-adapt in searching the optimal configuration during the training process. Hidden node (HN), iteration number (IN) and learning rate (LR) for both models are predetermined to be 100, 1000 and 1 respectively for comparison. Investigations were carried out with different population (b), maximum pulse frequency (fmax) and velocity factor (α). Models performance will be measured with Square Root of Correlation of Determination (r), Root Mean Square Errors (RMSE), Mean Absolute Error (MAE) and Nash and Sutcliffe coefficient (E). Data from 1961 to 1990 are used for training, whilst validation data are from 1991 to 2010. Predictors of three climate models including HadCM3, ECHAM5 and HadGEM3-RA cum collected precipitation data from Kuching Airport Rainfall Station are input into the models. Model output is the forecasted precipitation. Results showed BatNN is more robust than BANN with its average r = 0.96, average RMSE = 1.69, average MAE = 1.4 and average E = 0.84 across the three climate models; while BANN achieved average r = 0.95, average RMSE = 1.91, average MAE = 1.75 and average E = 0.82 across the three climate models. The higher accuracy of BatNN can be attributed to the modifications done where dynamical parameters R and A are used in place of static parameter to allow BatNN to self-adapt during the training process.