
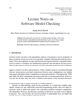
One way to recognize human emotions is to use physiological signals. In particular, EEG is noticed because it is non-invasive and inexpensive. However, it is difficult to perform recognition with high accuracy because there are a number of problems such as EEG signals have a lot of noise. The high accuracy analysis of EEG is the subject of research by many researchers. In this paper, we propose converting EEG signals into images and performing emotion classification tasks using CNN. In the experiment, we use DEAP dataset, which is often used in emotion recognition tasks using EEG. The EEG signal is divided into short segments based on a predetermined time window and plotted in time series data format to generate images. About the data plotting method, the image is generated by the method of making 32 classes and the method of making 4 classes. The generated images are classified into each emotions using a convolutional neural network. The classification use two axes, arousal and valence. The best results differ by gender. Men are able to get the best results when the time window is 1.0 with a 4-class image. The accuracy at these results is 63.75% for arousal and 63.36% for valence. The time window is 1.5 seconds and arousal is 65.37% when women use 4-class images. On the other hand, valence is 59.96% in 1.5 seconds when using a 32-class image. Also, it is found that arousal tends to be higher for women and valence tends to be higher for men. The experimental results show that the proposed method outperforms some related work. The proposed method is not dependent on the dataset, so it can be applied to research using various data.