
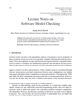
Flexible subsea risers are highly complex structures with multiple layers and varying material types. They are being used worldwide for oil and gas extraction in deep water. The complexity of these flexible risers and the hostile conditions in a deep-water environment present major challenges for non-intrusive, in-service inspection. This paper presents a novel automated radiography inspection system to detect defects from X-ray images of such flexible risers. The concept of a robotic digital X-ray scanning system which addresses the needs and challenges of deep-water flexible riser inspections has been studied. The proposed radiography inspection system is an ideal solution for flexible risers as it penetrates through all the layers in the riser structure, providing detailed information on any damage to the various layers. The system integrates the advanced technologies of image processing, machine learning, and robot crawlers to automatically detect abnormal textures such as erosion, corrosion and strand damage, foreign objects and other critical features from flexible risers. The performance of feature extraction, feature normalization, image processing, and machine learning for the purpose of defect detection have been tested and analysed. With smart integration and enhancement of different techniques, the limitations of each algorithms have been mitigated while the overall performance has been improved significantly. The detailed stages of the implementation are also discussed. The key features of the subsea riser inspection system are: (i) Real-time radiographic inspection result, (ii) Robotic system adaptable to various pipe types and sizes, (iii) High accuracy and reliability.