
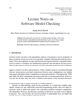
Cloud enabled systems have been increasingly adopted by manufacturing industries. The effectiveness of the cloud systems is, however, crippled by the high latency of data transfer between shop floors and cloud. To overcome the limitation, this paper presents an innovative fog based system for diagnosing and optimizing machining processes. The system includes: (1) dynamic diagnosis – Convolutional Neural Network (CNN) based diagnosis is implemented to detect potential faults from customized machining processes; (2) a fog enabled architecture for diagnosing and optimizing machining processes – it consists of a terminal layer, a fog layer and a cloud layer to minimize data traffic and improve system efficiency. Under the architecture, machining processes are monitored on the terminal layer and monitored signals are processed using the trained CNN deployed on the fog layer to efficiently detect abnormal situations. Intensive computing activities like training of the CNN and system re-optimization responding to detected faults are carried out dynamically on the cloud layer to leverage its computation powers. The system was validated in real-world production. In comparison with a cloud system, this fog system achieved 70.26% reduction in bandwidth between shop floors and cloud.