
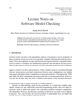
Precision agriculture adopts a set of techniques capable of increasing productivity, yield and efficiency in work related to agriculture, producing a greater benefit for farmers. In this study we focus on the problem of predicting weather conditions, specifically the prediction of low temperatures. Temperature prediction is a major problem in agriculture. Farmers can lose their crops if frost control techniques are not activated in time. The threshold for activating such techniques depends on the type of crop. A first preliminary study using deep learning is proposed to predict temperature, particularly a Long Short-Term Memory Network (LSTM) is used. The LSTM has been trained using real temperature data provided by an the Internet of Things (IoT) system, deployed in several plots and currently in operation. The results obtained after testing the model created with this neural network are quite satisfactory obtaining a determination coefficient (R2) of 99% and an average quadratic error of less than 0.8 degrees Celsius. Given the goodness of the model this can be implemented as an intelligent component of the IoT system, thus complementing its functionality.