
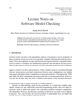
Many sophisticated methods exist to develop clinical decision support systems for daily clinical practice. In the core medical community, however, researchers often stick to basic methods due to lack of expertise. The International Ovarian Tumor Analysis (IOTA) study group, however, aims to explore advanced mathematical modeling options for ovarian tumor diagnosis through interdisciplinary collaborations involving clinicians, statisticians, and engineers. This resulted in several projects involving Bayesian models to distinguish between benign and adnexal ovarian tumors (binary classification). This chapter describes these projects. Major findings are that the classification of ovarian tumors appears to be a fairly linear problem, that benign and malignant tumors can be predicted with high accuracy, that complex black-box models can be further clarified using rule extraction, that input selection incorporating the cost of the available inputs leads to well-performing models with low total input cost, and that the widely used yet controversial and costly CA-125 tumor marker is not indispensable in mathematical diagnostic models. In conclusion, the interdisciplinary approach adopted by IOTA has resulted in useful clinical and technical insights concerning ovarian tumor diagnosis.