
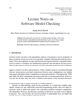
Passenger traffic volume as the basic data of traffic science management, has extremely high feedback ability and research value. The timely and accurate prediction of passenger traffic volume plays an important role in intelligent traffic management and control. With the rapid increase of traffic data volume in today society, along with highly nonlinear and random traffic systems, the prediction of passenger traffic volume is still a problem. This paper uses the long-term and short-term memory (LSTM) neural network, combined with actual passenger traffic data, through experiments and comparison with other representative forecast models validates that the proposed LSTM network can achieve a better performance, simultaneously analyzed the effects of various input settings on the LSTM forecast performance. In the passenger environment with large quantity and periodic regularity, the calculation time is saved and the prediction precision is improved, and the calculation speed of the same scale prediction problem is greatly improved. It can effectively provide the reference of traffic scheduling and planning during peak hours for the main transport stations with large passenger traffic.