
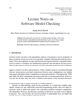
The main purpose of Automatic Speech Recognition systems consists of converting audio signals into text sequences in a reliable way. Most of these recent systems have been developed on English language. In the case of other languages such as Spanish, the development of these techniques is not sufficiently advanced due to the lack of properly transcriptions. As consequence, the implementation of a Spanish speech-to-text system arises as a complex and time-consuming task. Nevertheless, semi-supervised learning approaches can be suitable when low-amount of data supposes a barrier for building precise automatic speech recognition methods. Among the most remarkable approaches for solving the speech-to-text task, Deep Neural Networks have obtained outstanding results thanks to their ability for generalizing with smaller number of parameters in comparison to classical methods such as Gaussian Mixture Models. In this contribution, we propose a Spanish Automatic Speech Recognition system based on a semi-supervised learning approach, which uses Deep Neural Networks for codifying sounds into sequences of words. Hence, a Neural Network is trained in order to obtain an acoustic model. In the test phase, the sentences with the lowest error, following the Word Error Rate and the Fuzzy Match Score metrics, are included into the initial training dataset for re-training the acoustic model. Moreover, our proposal has been compared to a Gaussian Mixture Models-based approach. An improvement of 5% relative Word Error Rate was obtained. These results suggest that our technique obtains promising results by supporting in the task of building an Automatic Speech Recognition System when audio-transcription resources are scarce.