
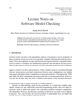
Cybersecurity has a lot of challenging problems, from intrusion to illegal actions and destruction. These challenges have attracted so many interests from researchers and practitioners in providing sustainable solutions. The presence of big data has increased the hope in curbing the aforementioned challenges due to its advantage of providing the platform for improved technology-based advancements. With the rapid development in the adoption of cloud-based services and migration of data to the cloud, there is a genuine need for advanced protection and prediction techniques. With this regard, Granular Computing is introduced as a new paradigm capable of providing solution to myriad of problems among which are related to cybersecurity. In this study, by taking the advantage of Granular Computing and Big Data, an improved k-means information granulation framework that incorporate similarity measure from the pre-clustering stage as well as segregation technique is proposed based on Granular Computing to identify threats from a time series dataset. The experiments on public available dataset shows that the method have good recognition performance better than other known predictive analysis classifiers – kNN and naive bayes. This study also provides research direction towards enhancing data granulation techniques in handling uncertainties.