
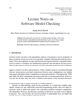
It's a world of full of information. Data is one of the important element of this era. One of the major sources of data is social media platforms like Twitter, Facebook etc. Everyday social media generates lot of data. It's a free form of communication where people communicate with each other without any restriction. Users can post anything on social media. However, human tendency of speaking about non-existing or source-less thing makes it unclear or create unuseful information and becomes unreliable information. This casual and word-of mouth form of communication leads to generate uncertain data and quality of information from factuality point of view becomes primary concern in social media. So, uncertainty detection is important in social media. Uncertainty detection in natural language text becomes challenging because dealing with natural language text is pretty complicated thing. Uncertainty is an important field of linguistics. Basically, it means “lack of information”. So, a statement whose truth value cannot be determined is considered as uncertain. Linguistics and Natural Language Processing aims at classifying factual and uncertain proposition. In this chapter, comparisons of classification algorithm have been experimented to detect uncertain propositions in Twitter data of food price crisis. Comparative analysis of classification algorithm Naive Bayes and Support Vector Machine approach is done to detect uncertain propositions of tweets related to food price crisis. A model is trained to classify certain or uncertain proposition using a training file which is annotated using cue words available in English language text. Output of algorithm is the class showing given proposition is certain or uncertain. The objective of this chapter is to have a comparative analysis of text classification approach to detect uncertain events of Twitter data of food price crisis and to improve the accuracy of uncertainty classification approaches in order to detect uncertain events in natural language processing.