
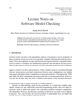
Outcomes research and evidence-based medical practice is being positively impacted by proliferation of healthcare databases. Modern epidemiologic studies require complex data comprehension. A new tool, DisEpi, facilitates visual exploration of epidemiological data supporting Public Health Knowledge Discovery. It provides domain-experts a compact visualization of information at the population level. In this study, DisEpi is applied to Attention-Deficit/Hyperactivity Disorder (ADHD) patients within Clalit Health Services, analyzing the socio-demographic and ADHD filled prescription data between 2006 and 2016 of 1,605,800 children aged 6 to 17 years. DisEpi's goals facilitate the identification of (1) Links between attributes and/or events, (2) Changes in these relationships over time, and (3) Clusters of population attributes for similar trends. DisEpi combines hierarchical clustering graphics and a heatmap where color shades reflect disease time-trends. In the ADHD context, DisEpi allowed the domain-expert to visually analyze a snapshot summary of data mining results. Accordingly, the domain-expert was able to efficiently identify that: (1) Relatively younger children and particularly youngest children in class are treated more often, (2) Medication incidence increased between 2006 and 2011 but then stabilized, and (3) Progression rates of medication incidence is different for each of the 3 main discovered clusters (aka: profiles) of treated children. DisEpi delivered results similar to those previously published which used classical statistical approaches. DisEpi requires minimal preparation and fewer iterations, generating results in a user-friendly format for the domain-expert. DisEpi will be wrapped as a package containing the end-to-end discovery process. Optionally, it may provide automated annotation using calendar events (such as policy changes or media interests), which can improve discovery efficiency, interpretation, and policy implementation.