
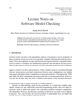
In this study, two major applications are introduced to develop advanced deep learning methods for credit card data analysis. Credit card information is contained in two data sets; credit approval dataset and card transaction dataset. The credit card dataset has two problems. One problem is using credit card approval dataset, it is necessary to combine multiple models, each referring to a different clustered group of users. The other problem is using card transaction dataset, since the actual unauthorized credit card use is very small, these imprecise solutions do not allow the appropriate detection of fraud. To solve these problems, we proposed deep learning algorithm to apply credit card dataset. The proposed methods are validated using benchmark experiments with other machine learnings. To evaluate our proposed method, we use two credit card datasets, credit approval dataset by UCI machine learning repository and credit transaction dataset constructed by random. The experiments confirm that deep learning exhibits comparable accuracy to the Gaussian kernel support vector machine (SVM). The proposed methods are also validated using large scale transaction dataset. Moreover, we apply our proposed method for the time-series benchmark dataset. Deep learning parameter adjustment is difficult. By optimizing the parameters, it is possible to increase the learning accuracy.