
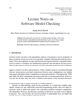
Diabetic Retinopathy is one of the main causes of blindness and visual impairment for diabetic population. The detection and diagnosis of the disease is usually done with the help of retinal images taken with a mydriatric camera. In this paper we propose an automatic retina image classifier that using supervised deep learning techniques is able to classify retinal images in five standard levels of severity. In each level different irregularities appear on the image, due to micro-aneurisms, hemorrages, exudates and edemas. This problem has been approached before using traditional computer vision techniques based on manual feature extraction. Differently, we explore the use of the recent machine learning approach of deep convolutional neural networks, which has given good results in other image classification problems. From a training dataset of around 35000 human classified images, different convolutional neural networks with different input size images are tested in order to find the model that performs the best over a test set of around 53000 images. Results show that it is possible to achieve a quadratic weighted kappa classification score over 0.75 not far from human expert reported scores of 0.80.