
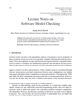
This paper analyzes and discusses the performance of Bag of Visual Words (BoVW), a well-known image encoding and classification technique utilized to recognize object categories, in the particular application scope of complex scene recognition. Given a set of training images containing examples of the different objects of interest, a dictionary of prototypical SIFT descriptors (visual words) is first obtained by applying unsupervised clustering. The contents of any input image can then be encoded by computing a histogram that denotes the relative frequency of every visual word in the SIFT descriptors of that input image. A Support Vector Machine (SVM) is then trained for every object category by using as positive examples the histograms corresponding to training images with objects belonging to that category, and as negative examples, the histograms from the other categories. Given an image with an unknown object to be classified, its histogram of visual words is obtained and fed into the available SVMs. The image is then classified into the object category of the SVM that yields the highest score. Although BoVW has proven to provide acceptable results for recognizing complex object categories, its performance for scene recognition needs to be assessed, since scenes have intrinsic properties that make them significantly differ from individual object categories.