
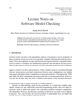
Automated video and image analysis can be a very efficient tool to behavior study, especially in hard access environments for researchers. The understanding of this social behavior can play a key role in the sustainable design of control policies of many species. This paper proposes the use of computer vision algorithms to identify and track, the Norway lobster, Nephrops norvegicus, a burrowing decapod with relevant commercial value which is captured by trawling. These animals can only be captured when are engaged in seabed excursions, which are strongly related with their social behavior. This emergent behavior is modulated by the day-night cycle, but social interactions remain unknown to the scientific community. The paper introduces an identification scheme made of four distinguishable black and white tags (geometric shapes). The project has recorded 15-day experiments in laboratory, under monochromatic blue light (472 nm.) and darkness conditions (recorded using Infra Red light). Using this massive image set, we propose a comparative of state-of-the-art computer vision algorithms to distinguish and track the different animals' movements. We evaluate the robustness to the high noise presence in the infrared video signals and free out-of-plane rotations due to animal movement. The experiments show promising accuracies under a cross-validation protocol, being adaptable to the automation and analysis of large scale data. In a second contribution, we created an extensive dataset of shapes (46027 different shapes) from four daily experimental video recordings, which will be available to the community.