
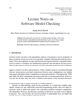
Background: In health care, exchange of data is becoming increasingly important. However, it is still unclear how to interpret measurements of the same parameter from diverse data sources. In clinical chemistry point-of-care testing (POCT), e.g. for blood glucose, complements the analytical spectrum of central laboratories. Available data from “central laboratory”, “professional POCT” and “non-professional” blood glucose measurements can be used to realistically simulate errors in different environments. The ability to classify the disease status or to detect a significant change based on these simulated measurements was assessed in several scenarios.
Methods: Patients were simulated with realistic “true values” of blood glucose and a “diseased” or “non-diseased” status. To further simulate a change of blood glucose values, a random difference was created and classified as “significant” or “non-significant”. Scientific literature was searched for accuracy and precision of blood glucose measurements in different environments. These values were used to calculate “measured values” from the “true values”. In scenarios where only measurements from the same environment were combined the area under the ROC curve (AUC) was used to express classifier performance. Balanced accuracy was used for scenarios with a combination of measurements from different environments. Situations with and without prior knowledge of the data source were simulated separately.
Results: AUC for the classification of disease status remained around 0.68 in all scenarios. Median AUCs for the detection of a significant change ranged between 0.89 in the central laboratory and 0.76 in the non-professional environment. For the combination of different measurement environments median balanced accuracies reached 0.63 for the classification of the disease status and differed little between scenarios. Median balanced accuracy to detect a significant change ranged from 0.81 to 0.72. When there was no prior knowledge about the additional data source it could not be used to determine an optimal decision boundary. In these cases, median balanced accuracy was approximately 0.02 lower.
Conclusion: These simulations highlight issues that are relevant beyond the combination of blood glucose values. Measurements conducted to help clarify one medical issue are often reused in other contexts. However not all data sources are equally suitable for all analyses. Therefore, when data from laboratory medicine is exchanged, accompanying information that helps to estimate reliability is critically important.