
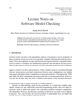
From the evolution of developing a pattern interestingness perspective, data mining has experienced two phases, which are Phase 1: technical objective interestingness focused research, and Phase 2: technical objective and subjective interestingness focused studies. As a result of these efforts, patterns mined are of significant interest to technical concern. However, technically interesting patterns are not necessarily of interest to business. In fact, real-world experience shows that many mined patterns, which are interesting from the perspective of the data mining method used, are out of business expectations when they are delivered to the final user. This scenario actually involves a grand challenge in next-generation KDD (Knowledge Discovery in Databases) studies, defined as actionable knowledge discovery. To discover knowledge that can be used for taking actions to business advantages, this paper addresses a framework that extends the evolution process of knowledge evaluation to Phase 3 and Phase 4. In Phase 3, concerns with objective interestingness from a business perspective are added on top of Phase 2, while in Phase 4 both technical and business interestingness should be satisfied in terms of objective and subjective perspectives. The introduction of Phase 4 provides a comprehensive knowledge actionability framework for actionable knowledge discovery. We illustrate applications in governmental data mining showing that the considerations and adoption of the framework described in Phase 4 has potential to enhance both sides of interestingness and expectation. As a result, knowledge discovered has better chances to support action-taking in the business world.