
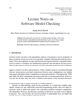
Predictive modeling in health care – from prediction to prevention For a number of decades, progress in medicine has relied on classical research pathways, which at some point involve the conducting of clinical trials. Ideally, a number of randomized clinical trials (RCTs) can be conducted and referred to in order to establish a high level of evidence for a given therapeutic intervention, be it a drug to be prescribed to the patient, a medical device to be used, or a particular health care process to be set-up.
RCTs are doubtless a very sound methodology for establishing both efficacy and safety, as well as cause-effect relationship, in an unambiguous way, but this process also has its limitations. Firstly, RCTs are expensive and lengthy procedures. At present, it seems that the number of potential innovative treatment options is developing much faster than can be assessed by a classical RCT approach in a reasonable amount of time. This is particularly the case in areas such as rare diseases. Secondly RCTs usually focus on a well characterized group of patients by means of proper inclusion and exclusion criteria. This can, however, significantly reduce the generalizability of the results to other groups such as the elderly, children, or patients with several co-morbidities. Thirdly, and most importantly, the trend towards precise and personalized medicine aims at the optimal approach for an individual patient, which presents a particular challenge for the classical approach to establishing evidence.
This is where predictive modeling comes in to play. Today, partly due to the increasingly digital nature of healthcare, vast amounts of data are available which can be used as a basis for profiling patient characteristics, to find out correlations between outcomes and potential predictors, and to predict future developments based on a plethora of existing cases.
In order to approach the concept of a truly personalized and preventive care plan tailored to the particular and complex needs of the individual patient, we need to include various sources of information, and predict the future course based on patient-specific models, as illustrated in Fig. 1. Ideally, such a model would not just tell us what could potentially happen to the health status of the individual, but also which options could best influence and modulate the person's situation so as to prevent unwanted events.
The question mark on the right hand side of Fig. 1 indicates that this is not an exhaustive list. There are many more potential sources of valuable information about an individual patient and his/her context which – taken into account – can help to tailor a healthcare strategy to the patient's particular needs.
Of course, correlations cannot be used to directly establish cause-effect relations, but what finally counts is whether a data driven approach works better than, or at least as well as, the state-of-the-art approach. And machine learning approaches usually improve when the amount of available data increases. This reflects the way in which humans become more experienced the more cases they have seen and managed. When it comes to “Big Data” environments however, the human mind faces limits in scalability which predictive modeling tools can potentially help to overcome.
Today, most predictive modeling approaches target non-medical goals in the fields of logistics and resource allocation, not least because predictive modeling concepts with a direct impact on patient treatment face huge regulatory hurdles. This seems to be a major factor in explaining why, so far, most of the data-driven predictive approaches directly related to patient care remain at a research level and in a retrospective setting. Only a few have made it into daily routine – but hasn't this initially been the case with almost all healthcare innovations? Nevertheless, we thought that now was the right time to make this topic “Predictive modeling in health care – from prediction to prevention” our theme for the 2016 issue of the annual scientific conference on “Health Informatics meets eHealth” in Vienna. It is time to give these new possibilities some additional visibility in the framework of the realm of eHealth as a whole.
Günter Schreier
Elske Ammenwerth
Alexander Hörbst
Dieter Hayn
March 19, 2016
Graz, Hall in Tirol, and Vienna