
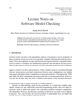
In this paper, we consider a typical health care system via the help of Wireless Sensor Network (WSN) for wireless patient tracking. The wireless patient tracking module of this system performs localization out of samples of Received Signal Strength (RSS) variations and tracking through a Particle Filter (PF) for WSN assisted by multiple transmit-power information. However, during the course of transmission power control, localization based on the RSS is a challenging problem because of the inconsistent RSS indication (RSSI) measurements in WSN. Therefore, we propose an adaptive-resampling, i.e. Kullback-Leibler Distance (KLD)-resampling with adjusted variance and gradient data, based on PF to improve the wireless patient tracking via smoothing down the effect of RSS variations by generating a sample set near the high-likelihood region for different transmit-power information. The key issue of this method is to use an adjusted variance and gradient data for reducing the wireless patient tracking error lower than 2 meter in 80% of the cases. We conduct a number of simulations using the health care's data set which contains real measurements of RSS collected to evaluate the accurate wireless patient tracking. The average wireless patient position error of our technique improves about 3% that of Sampling Important Resampling (SIR) PF, around 0.5% that of KLD-resampling, and about 6% that of gradient descent (i.e., unused PF). More importantly, when setting up suitable transmit-power level of self-RSSI, the simulation results show that the proposed technique outperforms these above existing methods, especial in the whole error from 0 to 3m. Finally, the simulation results also show the impact of number of particles for SIR algorithm, or the maximum number of samples for KLD-resampling algorithms on the wireless patient tracking when compared these traditional strategies in real experiment.