
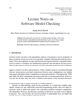
Trust is an essential necessity in Online Social Networks (OSNs) because of their current pervasive usage in sharing information on the Web. The traditional methods of measuring trust in social networks using heuristics or by manual methods are computationally expensive and sometimes do not offer reliable and scalable results. Recently, researchers resort to the use OSNs properties to model real life events because the interrelationships on these sites encode some human behaviors that resemble their off-line ones. Trust on these sites can, therefore, be measured by taking into consideration the social relationship and ties embeddedness within the underlying user graph. To this end, we study the distribution nature of “common friends”, the network mixing, average clustering coefficient and scale-free behaviors of 4 OSNs. The study shows that the distribution of “common friends” on OSNs follows a power law. Furthermore, to measure trust using these metrics, we proposed mathematical frameworks and analysis to support our claim. The findings show that these metrics have the ability to measure the level of trust on an unknown user on a social network of trust. These results will further help redefine and develop more reliable, fast and trust inference algorithms and recommender systems for online products and auction sales, email spam detection algorithms, etc. To the best of our knowledge, this work is the first to study the statistical distribution of “common friends” on OSNs and demonstrated how they can be exploited for trust inference.