
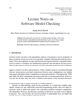
Recent research has been carried out along the line of multiple kernel methods for data fusion in self organizing maps and clustering, a vector quantization and a dimensionality reduction technique. However, the fixed grid size of Self-Organizing-Maps (SOM) knowing the nature of data in advance. This hinders the capabilities of SOM in various aspects (e.g. limits its applicability in complex data sets). This paper explores adaptation of multiple kernel methods for dynamically growing SOM, to adapt to variations in the distribution of the data. The primary focus has been application of kernel methods in the form of similarity measures while using the kernels in an optimal combination. This approach has been applied in the domain of road traffic visual information analysis. Several application specific video feature extraction techniques have been explored, based on recent research, to preprocess the video data in order to make it usable with the core algorithms. The inability to capture more useful knowledge from individual sources has been shown and successfully addressed by means of the fusion with multiple kernels. Individual performance of similar techniques in clustering are compared with the performance of this novel fusion approach. The experimental results convey the major improvements expected from the data fusion, including novelty detection and stability of results. Moreover, it signifies the necessity of heterogeneous data fusion in unsupervised learning to leverage complex real world data.