
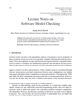
The general concept of the scientific method or procedure consists in systematic observation, experiment and measurement, and the formulation, testing and modification of hypotheses. In many cases a hypothesis is formulated in the form of a model, for example a mathematical or simulation model. The correctness of a solution of a problem produced by a model is verified by comparing it with collected data. Alternatively, observational data may be collected without a clear specification that the data could also apply to the solution of other, unforeseen problems. In such cases data analytics are used to extract relationships from and detect structures in data sets. In accordance with the scientific method, the results obtained can then be used to formulate one or more hypotheses and associated models as solutions for such problems. This approach allows for ensuring the validity of the solutions obtained. The results thus obtained may lead to a deeper insight in such problems and can represent significant progress in scientific research. The increased interest in so-called Big Data resulted in a growing tendency to consider the structures detected by analysing large data sets as solutions in their own right. A notion is thus developing that the scientific method is becoming obsolete. In this paper it is argued that data, hypotheses and models are essential to gain deeper insights into the nature of the problems considered and to ensure that plausible solutions were found. A further aspect to consider is that the processing of increasingly larger data sets result in an increased demand for HTC (High Throughput Computing) in contrast to HPC (High Performance Computing). The demand for HTC platforms will impact the future development of parallel computing platforms.