
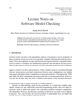
We consider the task of automatic classification of clinical incident reports using machine learning methods. Our data consists of 5448 clinical incident reports collected from the Incident Information Management System used by 7 hospitals in the state of New South Wales in Australia. We evaluate the performance of four classification algorithms: decision tree, naïve Bayes, multinomial naïve Bayes and support vector machine. We initially consider 13 classes (incident types) that were then reduced to 12, and show that it is possible to build accurate classifiers. The most accurate classifier was the multinomial naïve Bayes achieving accuracy of 80.44% and AUC of 0.91. We also investigate the effect of class labelling by an ordinary clinician and an expert, and show that when the data is labelled by an expert the classification performance of all classifiers improves. We found that again the best classifier was multinomial naïve Bayes achieving accuracy of 81.32% and AUC of 0.97. Our results show that some classes in the Incident Information Management System such as Primary Care are not distinct and their removal can improve performance; some other classes such as Aggression Victim are easier to classify than others such as Behavior and Human Performance. In summary, we show that the classification performance can be improved by expert class labelling of the training data, removing classes that are not well defined and selecting appropriate machine learning classifiers.