
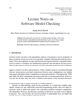
Multi-relational data mining is a rapidly growing area used for mining relational databases. While traditional data mining approaches search patterns in a single data table, relational data mining techniques look for patterns which exist in multiple tables. Multi-label learning (classification) comes under multi-relational data mining technique. In multi-label learning, each instance is linked with multiple labels and the aim is to predict the suitable label set for the unseen instance. In this paper, we reviewed the recent approaches for multi-relational data mining with a specific focus on multi-label classification. Furthermore, we critically evaluate the prevalent techniques for the prediction of class labels. Moreover, we propose a conceptual model for multi-label learning using label ranking and label correlation simultaneously. The proposed model works concurrently in two modules. In the first module, Label Ranking is used to enhance the classifier process by ranking the classes on the basis of priority and in the second module; degree of correlation of data labels among training classes has been utilized to enhance the overall process of multi-label learning.