
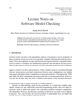
In this paper, we proposed a modified decision tree learning algorithm. In order to improve the traditional decision tree learning algorithm, we modified a predict phase though exists approached modified a learning phase. Our proposed approach makes a decision tree by a traditional decision tree learning algorithm and predicts new data items' class label by K-NN. The traditional decision tree learning algorithm predicts a class label based on the ratio of class labels in a leaf node. When it is not easy to classify data set according to class labels, leaf nodes includes a lot of data items and class labels. It causes to decrease the accuracy rate. However, it is difficult to prepare good training data set. So we used K-NN to predict a class label from data items in a leaf node. In order to evaluate our approach, we did an experiment using a part of open data sets from UCL learning repository. We compared our approach to ID3 which is one of traditional decision tree learning algorithms and K-NN in this experiment. Experimental result shows our approach is better than ID3 when the leaf nodes include a lot of data items. When the leaf nodes include some data items, our approach can perform like as ID3. So we can say that our approach is useful to modify a decision tree learning algorithm. We don't change a learning process so that our approach doesn't change the readability of a decision tree. In addition to, our approach is better than K-NN. We think that a decision tree works for K-NN as data cleaning. It says that our approach is useful for K-NN. Though we can show the advantage of our approach according to the experiment, there are some data items we can not predict correctly. In future, we have to evaluate experimental results and process in detail. We have to ascertain the cause of error. And we consider how to modify our approach to correct errors. It is likely that normalization is one of useful method. In addition to, we have to evaluate our new approach using some open data sets.