
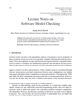
Patient safety is the buzz word in healthcare. Incident Information Management System (IIMS) is electronic software that stores clinical mishaps narratives in places where patients are treated. It is estimated that in one state alone over one million electronic text documents are available in IIMS. In this paper we investigate the data density available in the fields entered to notify an incident and the validity of the built in classification used by clinician to categories the incidents. Waikato Environment for Knowledge Analysis (WEKA) software was used to test the classes. Four statistical classifier based on J48, Naïve Bayes (NB), Naïve Bayes Multinominal (NBM) and Support Vector Machine using radial basis function (SVM_RBF) algorithms were used to validate the classes. The data pool was 10,000 clinical incidents drawn from 7 hospitals in one state in Australia. In first part of the study 1000 clinical incidents were selected to determine type and number of fields worth investigating and in the second part another 5448 clinical incidents were randomly selected to validate 13 clinical incident types. Result shows 74.6% of the cells were empty and only 23 fields had content over 70% of the time. The percentage correctly classified classes on four algorithms using categorical dataset ranged from 42 to 49%, using free-text datasets from 65% to 77% and using both datasets from 72% to 79%. Kappa statistic ranged from 0.36 to 0.4. for categorical data, from 0.61 to 0.74. for free-text and from 0.67 to 0.77 for both datasets. Similar increases in performance in the 3 experiments was noted on true positive rate, precision, F-measure and area under curve (AUC) of receiver operating characteristics (ROC) scores. The study demonstrates only 14 of 73 fields in IIMS have data that is usable for machine learning experiments. Irrespective of the type of algorithms used when all datasets are used performance was better. Classifier NBM showed best performance. We think the classifier can be improved further by reclassifying the most confused classes and there is scope to apply text mining tool on patient safety classifications.