
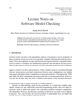
The aim of this study was to predict further cognitive decline in mild cognitive impairment (MCI) using an individual level support vector machine (SVM) classification analysis of white matter derived from diffusion tensor imaging (DTI). Thirty-five healthy controls (HC) and 67 MCI subjects had DTI at baseline. MCI subjects were neuropsychologically followed for after one year and categorized into 40 stable (sMCI; 9 single domain amnestic, 7 single domain frontal, 24 multiple domain) and 27 progressive (pMCI; 7 single domain amnestic, 4 single domain frontal, 16 multiple domain). Fractional anisotropy (FA), longitudinal (LD), radial (RD) and mean (MD) diffusivity were assessed using Tract-Based Spatial Statistics (TBSS). Statistical analyses included both group comparisons and individual classification using SVM using 10 fold cross validation. FA was significantly higher in HC compared to MCI in a distributed network including the ventral part of the corpus callosum, right temporal and frontal pathways. There were no significant group-level differences between sMCI versus pMCI or between MCI subtypes after correction for multiple comparisons. SVM analysis allowed for an individual classification with accuracies up to 91.4% (HC versus MCI) and 98.4% (sMCI versus pMCI). When considering the MCI subgroups separately, the minimum SVM classification accuracy for stable versus progressive cognitive decline was 97.5% in the multiple domain MCI group. SVM analysis of DTI data provided highly accurate individual prediction of cognitive decline in MCI regardless of MCI subtype, indicating that this method may become an easily applicable tool for early individual detection of MCI subjects evolving to dementia.