
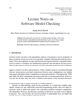
Scientific computing deals with large-scale scientific modelling and simulation in different domains like astrophysics, climate research, mechanical engineering and bio-informatics. Execution of large and accurate simulations in these domains requires significant computing resources. As such, scientific computing has always been closely connected to High Performance Computing (HPC) and Distributed Systems, utilising the computing resources of supercomputers, computer clusters and grids to perform the large scale calculations needed. Cloud is not different from it's predecessors as a resource for computing infrastructure, but provides even easier access to public resources with the increasing popularity of cloud computing and the success of many Infrastructure as a Service (IaaS) cloud providers, who rent out virtual infrastructure as utility. Cloud computing frameworks like MapReduce provide tools for implementing algorithms and can automatically parallelise them, which can greatly simplify the work of researchers. But MapReduce is focused on huge data processing and is less suitable for complex algorithms of scientific computing. We studied using different frameworks based on the MapReduce model for scientific computing and compared them to other distributed computing frameworks. In the process we explain our motivation for designing a new distributed scientific computing framework and the considered preliminary design choices.