
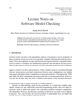
With the developments in the information technology and improvements in the communication channels, fraud is spreading all over the world, resulting in huge financial losses. Though fraud prevention mechanisms such as CHIP&PIN are developed for credit cards, these mechanisms do not prevent the most common fraud types such as fraudulent credit card usages over virtual POS terminals or mail orders so called online credit card fraud. As a result, fraud detection is the essential tool and probably the best way to stop such fraud types. In this study, classification models based on well-known data mining algorithms such as decision trees, Artificial Neural Networks (ANN), Logistic Regression (LR) and Support Vector Machines (SVM) are developed and applied on the credit card fraud detection problem. Furthermore, a new cost-sensitive decision tree algorithm that minimizes the sum of misclassification costs while selecting the splitting attribute at each non-terminal node of the tree is developed. Performances of the models developed are compared with respect to not only a well-known performance metric True Positive Rate (TPR), but also newly defined cost based performance metrics specific to the problem domain. The performance of the model built using this cost-sensitive decision tree algorithm in identifying frauds is compared with the pre-built models. The results show that this cost-based decision tree algorithm outperforms the existing well-known methods in terms of the fraudulent transactions identified and the amount of losses recovered. This method can be readily applied to real-world credit card fraud detection tasks.