
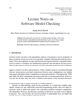
Text summarization is the process of distilling the most important information from source/sources to produce an abridged version for a particular user/users and task/tasks. Automatically generated summaries can significantly reduce the information overload on intelligence analysts in their daily work. Moreover, automated text summarization can be utilized for automated classification and filtering of text documents, information search over the Internet, content recommendation systems, online social networks, etc.
The increasing trend of cross-border globalization accompanied by the growing multi-linguality of the Internet requires text summarization techniques to work equally well on multiple languages. However, only some of the automated summarization methods proposed in the literature can be defined as “multi-lingual” or “language-independent,” as they are not based on any morphological analysis of the summarized text.
In this chapter, we present a novel approach called MUSE (MUltilingual Sentence Extractor) to “language-independent” extractive summarization, which represents the summary as a collection of the most informative fragments of the summarized document without any language-specific text analysis. We use a Genetic Algorithm to find the best linear combination of 31 sentence scoring metrics based on vector and graph representations of text documents. Our summarization methodology is evaluated on two monolingual corpora of English and Hebrew documents, and, in addition, on a bilingual collection of English and Hebrew documents. The results are compared to 15 statistical sentence scoring methods for extractive single-document summarization found in the literature and to several state-of-the-art summarization tools. These bilingual experiments show that the MUSE methodology significantly outperforms the existing approaches and tools in both languages.