
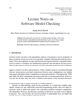
Bayesian networks (BNs) were pioneered to solve problems in Artificial Intelligence (AI) and have proven successful in “intelligent” applications such as medical expert systems, speech recognition, and fault diagnosis. In practical terms, one of the major benefits from using BNs is in that probabilistic and causal relationships among variables are represented and executed as graphs and can thus be easily visualized and extended, making model building and verification easier and faster. We illustrate how BNs can be used for risk analysis by introducing a novel approach modeling causal chains containing event triggers, consequences and interventions. However, if we want to incorporate continuous (as opposed to just discrete) variables in BN models the established BN tools and methods are inadequate. This paper reports on a new, unifying, approach to modeling continuous variables in BNs, called dynamic discretization, which approximates continuous variables without recourse to the traditional approach of Monte Carlo simulation methods. We illustrate the practical usefulness of the approach with an application involving the fusion of diverse sources of temporal data for fault diagnosis, classification and prediction of system behavior.