
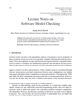
Accurate and consistent characterization of flawed regions in steam generator tubing is becoming increasingly important as nuclear power plants age and repair costs increase. The general problem of assessing the structural integrity of steam generator tubing using eddy current inspection techniques is rather complex due to the presence of noise and interfering signals under different inspection conditions. Additional difficulties in the data analysis task arise due to the fact that unwanted signals from support structures, probe wobble and geometry variations result are often similar to defect signals, thereby frustrating the characterization process. Automated analysis of eddy current inspection data can help in enhancing the accuracy and consistency of signal interpretation. This, in turn, offers significant benefits to utilities, including cost savings by reducing manpower needs, time savings by reducing the impact of analyst shortages during peak outage periods, providing analysis results at rates equal to or less than the rates for data acquisition, and reliability improvements by providing consistent, repeatable and accurate inspection results.
An overview of signal processing techniques for classification and characterization of defects in SG tubes along with typical implementation results will be presented. The automated signal analysis system provides utility engineers with detailed information on the dimension, shape, orientation, origin, and location of individual flaws within steam generator tubes. Such information can be employed by computational models for calculating burst and safe operating pressures.
Another tool that can help in improving inspection reliability is the availability of simulation models. The models enable visualization of field/flaw interaction, and better understand the effect of various geometrical and experimental parameters on flaw signals. A simple axisymmteric model for SG geometry with a user friendly interface can help in training operators. Such models can be employed to build probability of detection (POD) models capable of quantifying the capability of an inspection system.