
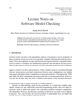
Essential for a valid analysis of treatment efficacy is a prospectively specified analysis, that is robust against violation of those assumptions, which cannot be ascertained a priori. Additional modelling for inference of the treatment effect makes sense, if it is intended to check validity and efficiency of the preplanned analysis and the influence of missing data and protocoll violations on the results. Secondly, the temporal course of therapeutic effect can be investigated and thirtly, a subgroup analysis may be done in order to search for variables that modify the therapeutic effect.
Unfortunately, the statistical modelling process is not easily planned prospectively. Hence for a balanced judgement it is recommended, to list all models eventually fitted, and weight them subjectively with respect to deemed validity and efficiency. The additional modelling should result in a statement about the preplanned analysis as to the validity of the effect estimate and and the efficiency of the procedure producing the corresponding p-value or confidence interval.
Using data of a study by Koscielny & al. on the efficacy of garlic on longterm reduction of artherosclerosis all three points are adressed by the way of Bayesian analysis of linear mixed models. Results are compared to those obtained by restricted maximum likelihood method.
It can be illustrated that posterior based tests and confidence intervals that belong to uninformative priors, are frequently very similar to classic confidence intervals and p-values. Hence they are interpretable within the categories of classical inferential statistics.
Where REML-Analysis is valid asymptotically only, it can be validated via Bayesian analysis. Further advantages are easy and robust way of handling missing values, a large collection of tractable models and the use of posterior densities as an intuitive add on to confidence intervals.