
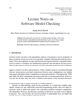
Magnetic Resonance (MR) imaging is a medical technique which permits the visualization of a variety of tumors, lesions, and abnormalities present within the soft biological tissues of the body. Segmentation of medical image data is the process of assigning anatomically-meaningful labels to each component of the image. This paper presents a method of knowledge-based interpretation of MR images of the head. In particular, the method is designed for the segmentation, or detection, of multiple sclerosis (MS) lesions of the brain. Knowledge of brain anatomy is represented in the form of a tissue probability model which provides a priori probabilities of brain tissue distribution per unit voxel in a standardized 3D ‘brain space’. As 90-95% of 118 lesions occur in white matter tissue [1], the model was used to confine the search for MS lesions to plausible white matter locations. Use of the model to segment multiple sclerosis lesions reduced the number of false positive lesions by 50-80% in comparison to non-model-based (i.e. purely data-driven) segmentation.
The performance of the statistical minimum distance and Bayesian classifiers (which both represent learned tissue classification rules as mathematical formulae) are compared to that of pruned and unpruned decision tree classifiers (which represent classification rules symbolically, as flowchart-like tree structures). Although each classifier performed at about the same level of accuracy, the concise and symbolic representation of the rules generated by the pruned decision tree classifier may be preferred for its greater human interpretability.