
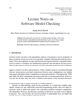
Edgar Wingender
Department of Bioinformatics, University Medical Center Göttingen, Göttingen, Germany, E-mail: e.wingender@med.uni-goettingen.de
As “classical” bioinformatics developed further towards modern systems biology, the idea of a holistic view of a biological system was not completely new: the aim to provide a comprehensive picture of genes and the regulatory features encoded in a genome had been inherent in bioinformatics research from the very beginning, as was the attempt to come up with an integrative view across the different levels of organisation, which was at least conceptually implicit in the numerous approaches to integrating the rapidly growing information about biological objects into comprehensive knowledge bases. However, transcendence of the research focus on static objects and progress towards the computer-aided investigation of biological processes was significantly advanced by the emerging field of systems biology. The new paradigm for formally representing the processes that make up a biological system is now the “network”.
The term “process” itself implies dynamic events, changes, that we may wish to simulate with the aid of a computer in order to predict the behavior of a biological system under certain circumstances. Biochemistry provides the formal instruments to do so for defined (bio)chemical reactions, usually resulting in a set of ordinary differential equations (ODEs). Solving the large number of ODEs that are required to describe the behavior of a complex biological system exactly may be cumbersome, but computationally feasible as soon as we have at hand all the necessary parameters, such as the corresponding kinetic constants for all reactions involved. Even in those cases where these kinetics have been studied in vitro, it is still questionable whether the insights gained from these experiments are applicable to specific in vivo conditions. Nevertheless, this approach has been proven to work for (parts of) the metabolic network of living cells, but regulatory events that depend on a very low number of individual molecules per cell may require different approaches. Moreover, applying ODEs to a large complex system may be mere overkill, and, presumably, a less exact approach might be of even more appropriate granularity, at least for the larger part of the network under consideration.
Several years ago, Petri nets were suggested as being well suited to modeling metabolic networks, overcoming some of the limitations outlined above [Reddy et al., 1993]. Since then, a great deal of further conceptual work, technical tool implementations and applications to biological problems have been reported which have demonstrated the usefulness of this concept for what we know today as systems biology. Being intuitively understandable to scientists trained in the life sciences, they also have a robust mathematical foundation and provide the required flexibility with regard to the models' granularity. As a result, Petri net technology appears to be a very promising approach to modeling biological systems.
A significant part of the progress in this field has been published by In Silico Biology since it began in 1998. Four articles constituted the first Petri Net Special in 2003 (“Petri Nets for Metabolic Networks”; http://www.bioinfo.de/isb/toc_vol_03.html). R. Hofestädt's introduction to that Special Issue summarized the most essential topics as well as some of the basic requirements and constraints relevant for applying Petri net technology, and it therefore follows this preface [Hofestädt, 2003].
The early publication of Hofestädt and Thelen, 1998, demonstrated how the Petri net concept can be extended to a more quantitative modeling of metabolic networks, and also expanded these ideas to gene regulatory and cell-cell communication processes. In a subsequent work,Chen and Hofestädt were able to demonstrate how the Petri net approach can deal with an integrated process comprising gene regulatory and metabolic events, making use of the concept of hybrid Petri nets (HPN) [Chen and Hofestädt, 2003]. Having analyzed the requirements of the biochemical particularities of metabolic networks, Zevedei-Oancea and Schuster, 2003, studied the resulting topological properties of the corresponding Petri net models. Takai-Igarashi suggested a consistent definition of the Petri net units that are required for modeling signal transduction pathways; this was based on a specific ontology, the Cell Signaling Networks Ontology (CSNO) [Takai-Igarashi, 2005]. Voss et al., 2003, complemented these efforts by studying the Petri net models of metabolic steady states including all relevant reverse reactions. Similarly, Gambin et al. made an attempt to model the stationary state of a gene regulatory network in a Petri net [Gambin et al., 2006]. A big step forward towards dynamic simulation with the aid of Petri net models was undertaken by Matsuno et al., 2003, by extending the underlying approach further to the concept of hybrid functional Petri nets (HFPN). It was proved that this could be applied to simulating the dynamics of two regulatory pathways, and it was demonstrated how such a Petri net model could be constructed [Matsuno et al., 2003; Doi et al., 2004]. More recently, the concept of firing delay times was introduced into the HFPN approach and applied to a signaling process [Miwa et al., 2010].
The conceptualization phase was also accompanied by the active development of a number of tools assisting in the creation and manipulation of biological Petri nets. While the early efforts used a platform that was originally developed for technical purposes (Visual Object Net ++, VON++) [Chen and Hofestädt, 2003], a tool specifically developed soon afterwards for modeling biological processes with Petri nets was published: Genome Object Net, GON [Matsuno et al., 2003; Doi et al., 2004]. GON evolved subsequently into Cell Illustrator (CI), a platform that is suitable for easy modeling and simulating the dynamics of cellular processes [Nagasaki et al., 2010]. A specialized tool (STEPP) for searching in a Petri net for those paths that connect, e.g., two metabolites and thus generating hypotheses about the interconversion of these two compounds was introduced by Koch et al., 2004; the program is still available for download (http://www.bioinformatik.uni-frankfurt.de/tools/stepp/stepp.html). Janowski et al. developed a powerful network editor, VANESA, that is able to work with Cell Illustrator as a simulation engine [Janowski et al., 2010].
A number of applications of Petri net-based modeling and simulation have been published in recent years. While early work usually focused on certain parts of the metabolic network such as glycolysis and pentose phosphate pathway [Voss et al., 2003; Zevedei-Oancea and Schuster, 2003; Doi et al., 2004], nucleotide metabolism [Zevedei-Oancea and Schuster, 2003], urea cycle [Chen and Hofestädt, 2003], or the conversion of sucrose into starch in potato [Koch et al., 2004], different kinds of regulatory networks have attracted more attention of late. Thus, several signal transduction networks have been studied in great detail: the Fas ligand-induced cascade leading to apoptosis [Matsuno et al., 2003], the TGF-beta pathway [Takai-Igarashi, 2005], and the p53 network [Doi et al., 2006]. This year, the IL-1 pathway was added [Miwa et al., 2010].
Gene regulatory events have also been studied, such as the control of circadian rhythm in Drosophila [Matsuno et al., 2003], the regulation of glycolysis by the lac operon [Doi et al., 2004], the flower morphogenesis of Arabidopsis [Gambin et al., 2006]. The latter topic has now been resumed by Kaufmann et al., with the aid of Cell Illustrator [Kaufmann et al., 2010]. The mRNA turnover for a number of components relevant for cell-cycle regulation was studied with a stochastic Petri net by Csikász-Nagy and Mura, 2010. The assembly of the splicesosome has been modeled by Bortfeldt et al., 2010, analyzing the modular nature of this regulatory network.
How intercellular communication processes can be linked with intracellular regulatory events and simulated has been demonstrated in the contributions of Janowski et al., taking the bacterial quorum sensing as an example [Janowski et al., 2010]. The impact of delays and noise on the dopamine signal transmission was investigated by E. Voit and colleagues [Wu et al., 2010], and both studies made use of the HFPN-base simulation engine of Cell Illustrator [Nagasaki et al., 2010].
This recent overview was published as a Special Issue on Petri Net Applications in Molecular Biology of ISB volume 10, and the entire collection now constitutes this First ISB Book on Biological Petri Nets. We are confident that the reader will benefit from this unique compilation of articles, and hope that it helps to illustrate the value of the Petri Net approach to modern life sciences.
Sadly, on the 2nd of July, 2010, while this book was in the last stages of editing, Prof. Dr. Carl Adam Petri passed away. We mourn the loss of a great scientist. His work has inspired researchers from a broad range of disciplines, which clearly indicates his perspicacious mindset. We would like to honor his outstanding scientific merits by dedicating this book to his memory.
REFERENCES
• Bortfeldt, R. H., Schuster, S. and Koch, I. (2010). Exhaustive analysis of the modular structure of the spliceosomal assembly network - a Petri net approach. In Silico Biology 10, 0007.
• Chen, M. and Hofestaedt, R. (2003). Quantitative Petri net model of gene regulated metabolic networks in the cell. In Silico Biology 3, 0030.
• Csikász-Nagy, A. and Mura, I. (2010). Role of mRNA gestation and senescence in noise reduction during the cell cycle. In Silico Biology 10, 0003.
• Doi, A., Fujita, S., Matsuno, H., Nagasaki, M. and Miyano, S. (2004). Constructing biological pathway models with hybrid functional Petri net. In Silico Biology 4, 0023.
• Doi, A., Nagasaki, M., Matsuno, H. and Miyano, S. (2006). Simulation based validation of the p53 transcriptional activity with hybrid functional Petri net. In Silico Biology 6, 0001.
• Gambin, A., Lasota, S. and Rutkowski, M. (2006). Analyzing stationary states of gene regulatory network using Petri nets. In Silico Biology 6, 0010.
• Hofestädt, R. (2003). Petri nets and the simulation of metabolic networks. In Silico Biology 3, 0028.
• Hofestädt, R. and Thelen, S. (1998). Quantitative modeling of biochemical networks. In Silico Biology 1, 0006.
• Janowski, S., Kormeier, B., Töpel, T., Hippe, K., Hofestädt, R., Willassen, N., Friesen, R., Rubert, S., Borck, D., Haugen, P. and Chen, M. (2010). Modeling of cell-cell communication processes with Petri nets using the example of quorum sensing. In Silico Biology 10, 0003.
• Kaufmann, K., Nagasaki, M. and Jáuregui, R. (2010). Modelling the molecular interactions in the flower developmental network of Arabidopsis thaliana. In Silico Biology 10, 0008.
• Koch, I., Schüler, M. and Heiner, M. (2004). STEPP – Search Tool for Exploration of Petri net Paths: A new tool for Petri net-based path analysis in biochemical networks. In Silico Biology 5, 0014.
• Matsuno, H., Tanaka, Y., Aoshima, H., Doi, A., Matsui, M. and Miyano, S. (2003). Biopathways representation and simulation on hybrid functional Petri net. In Silico Biology 3, 0032.
• Miwa, Y., Li, C., Ge, Q.-W., Matsuno, H. and Miyano, S. (2010). On determining firing delay time of transitions for Petri net based signaling pathways by introducing stochastic decision rules. In Silico Biology 10, 0004.
• Nagasaki, M., Saito, A., Jeong, E., Li, C., Kojima, K., Ikeda, E. and Miyano, S. (2010). Cell Illustrator 4.0: A computational platform for systems biology. In Silico Biology 10, 0002.
• Reddy, V. N., Mavrovouniotis, M. L. and Liebman, M. N. (1993). Petri net representation in metabolic pathways. Proc. Int. Conf. Intell. Syst. Mol. Biol. 1, 328-336.
• Takai-Igarashi, T. (2005). Ontology based standardization of Petri net modeling for signaling pathways. In Silico Biology 5, 0047.
• Voss, K., Heiner, M. and Koch, I. (2003). Steady state analysis of metabolic pathways using Petri nets. In Silico Biology 3, 0031.
• Wu, J., Qi, Z. and Voit, E. O. (2010). Impacts of delays and noise on dopamine signal transduction. In Silico Biology 10, 0005.
• Zevedei-Oancea, I. and Schuster, S. (2003). Topological analysis of metabolic networks based on Petri net theory. In Silico Biology 3, 0029.